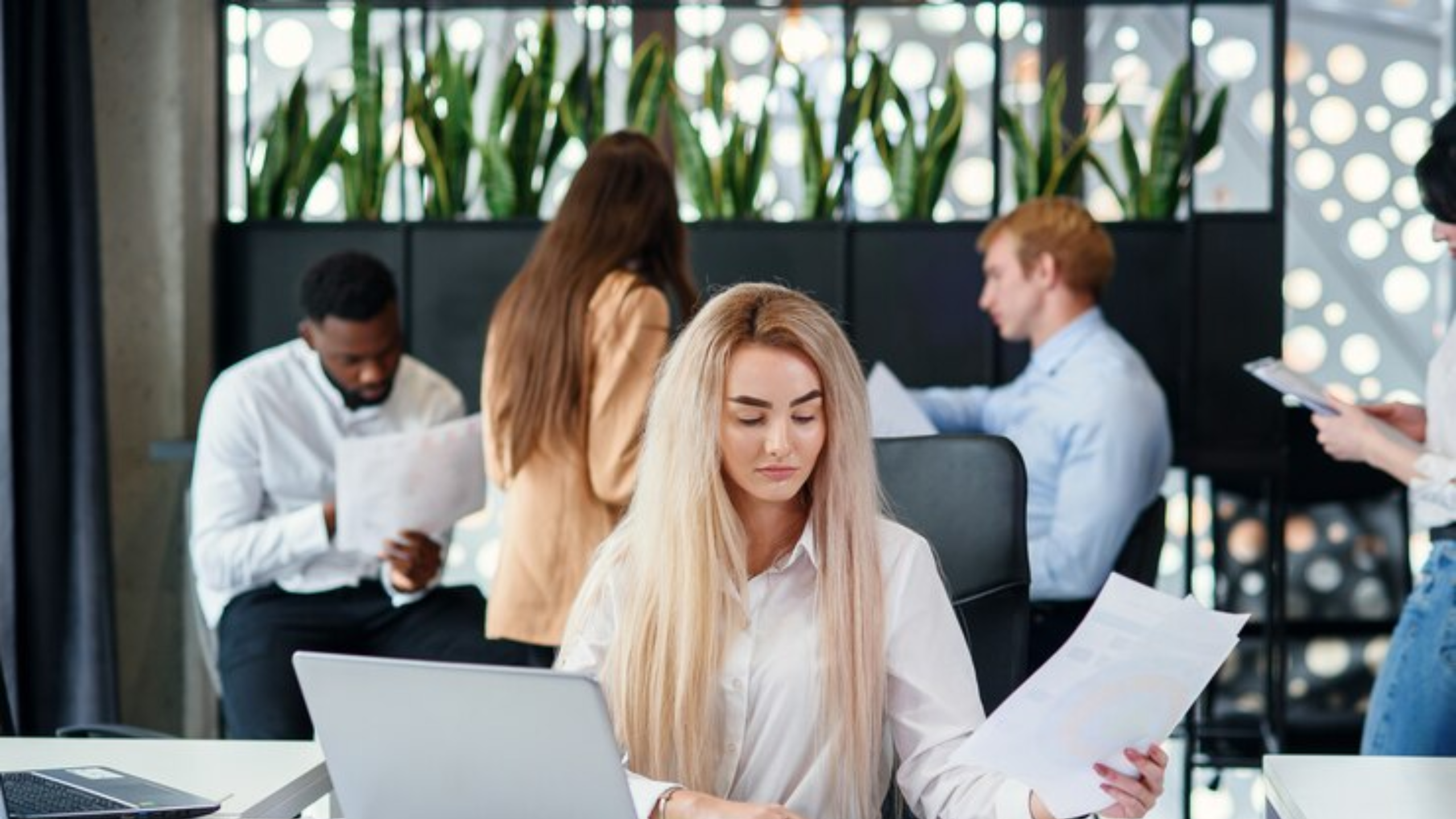
In our previous post How To Design A Systematic Strategy, we discussed the various elements that go into making a systematic strategy, here we shall look at the data component in a little more detail and try to understand various data sources and the practical aspects we need to be mindful of before using them. For the purpose of this post, we shall reference data in the context of the factors/independent variables/input data that are used to generate the trading decision or signal. While there could be multiple data choices for what we are trying to estimate/predict, in most cases this tends to be returns, either directly or indirectly. We shall also use the term ‘predict’ interchangeably for an estimation or decision.
Types of Input Factors
While the choice of the input data can seem daunting, a good way to narrow this down is based on a) type of strategy, b) asset classes involved and c) duration of prediction. For instance, if the strategy is to allocate capital across multiple asset classes over the medium to long term, we would require macroeconomic factors and an understanding of relative drivers for each asset class. There are different metrics that are relevant based on the asset being traded- stocks have earnings data and analyst recommendations, corporate debt has credit ratings and yields and commodities have inventories data. Similarly, the duration of the prediction could rule out some factors, for e.g., a high frequency trading strategy on stocks is unlikely to use earnings or economic data while price and volumes will be relevant.
Macroeconomic Factors:
Factors that quantify or point to the state of the economy or the state of macroeconomic variables (GDP growth, inflation etc) would be classified as macro factors. These could either be local factors like country growth rate, current account balances, domestic inflation or global factors like oil prices and equity risk sentiment. The data here could include indicators of the health of the financial markets, monetary conditions, real economy, price pressures, unemployment or any such other economic factor. Such factors are relevant to all asset classes and are often heavily used in multi asset strategies, where capital allocation needs to shift quickly between different assets. Several index providers also create macroeconomic indices which aggregate different kinds of data into a consolidated score for comparison and ease of use.
Core Factors:
These are the set of factors, based on which most individual instruments would be priced off, given a theoretical pricing framework; think earnings statements for stocks, credit ratings for corporate bonds or demand-supply for commodities. These could also loosely be classified as fundamental factors, although the term probably includes a lot more information that is not always quantifiable. Most equity portfolios lean heavily on analyzing the health of companies through financial statements and the breadth of data available in such reports is enormous. Analysts often combine insights from data across the balance sheet, profit and loss statement and the cash flow statement. Since most of these factors are generally available at a quarterly or lower frequency, these are generally more suited to medium to high duration strategies.
Traded Data:
For any tradeable security, this includes all the variables that are available from the venues/exchanges they trade at for e.g. price, yield, volume, open interest, delivery volumes, spot-futures basis etc. For exchange traded securities, this could include granular data from the order book or even tick and order level data, which is where most high frequency strategies operate. The way prices move, also called price action, is a huge area of active analysis and several strategies rely on price and its derived indicators alone. Technical patterns or chart analysis almost entirely relies on price and volume data and these have been implemented across asset classes. Since most of these variables are available real time or with a day’s lag, they can be modified to be used across strategy durations.
Ownership & Flows:
Since most securities, barring commodities, are financial instruments, the amount and level of ownership of different categories of investors and the relative shifts could have some influence on the nature of price moves. For instance, if foreign institutional investors are the largest holders of a particular bond or stock, then their selling could precipitate a large fall in price. On the other hand, if a particular security has very few institutional investors, it could be seen as a high-risk bet given the absence of larger players. Ownership patterns are easily available for listed stocks through exchanges but may not be as straight forward for other asset classes, although most financial regulators do share such information at frequent intervals. These data sets are generally used in conjunction with other data sources and are very rarely used standalone.
Sentiment Factors:
While most of the above factors generally capture tangible measures and variables, often financial markets are driven by emotions and greed and fear alone. Moves in one market could lead to knock on effects in other seemingly unrelated markets and this has happened more than once in our recent past. Sentiment factors are the broad set of indicators and measures that people use to gauge whether the underlying mood of the markets is one of optimism or pessimism. There is no standard definition of what kind of variables can be used here, but some examples are underlying market activity, options data, analysts’ expectations and consumer or business confidence.
Alternative Data:
Any data source that is not traditionally used or has very few users can technically be classified here. The significant computing power available to us has made big data processing a reality and several sophisticated investors are now increasingly augmenting their existing factors with such datasets to corroborate or challenge their hypotheses. Satellite data imagery has been used by some institutional investors to identify movement of ships across the seas or amount of night time light in industrial areas as a proxy for demand-supply activity. Credit card and retail spends can also be used in a similar way and can be a lead indicator for consumer optimism or cautiousness. While some of these could be publicly available, most are proprietary and involve significant costs.
Some strategies might just use a single kind of data while others might use more, this is purely a design choice and depends on the purpose and mandate of the strategy. In our next post, we shall look at some of the practical aspects we need to be mindful of before using some of the above datasets.